Google’s MedPaLM emphasizes human clinicians in medical AI
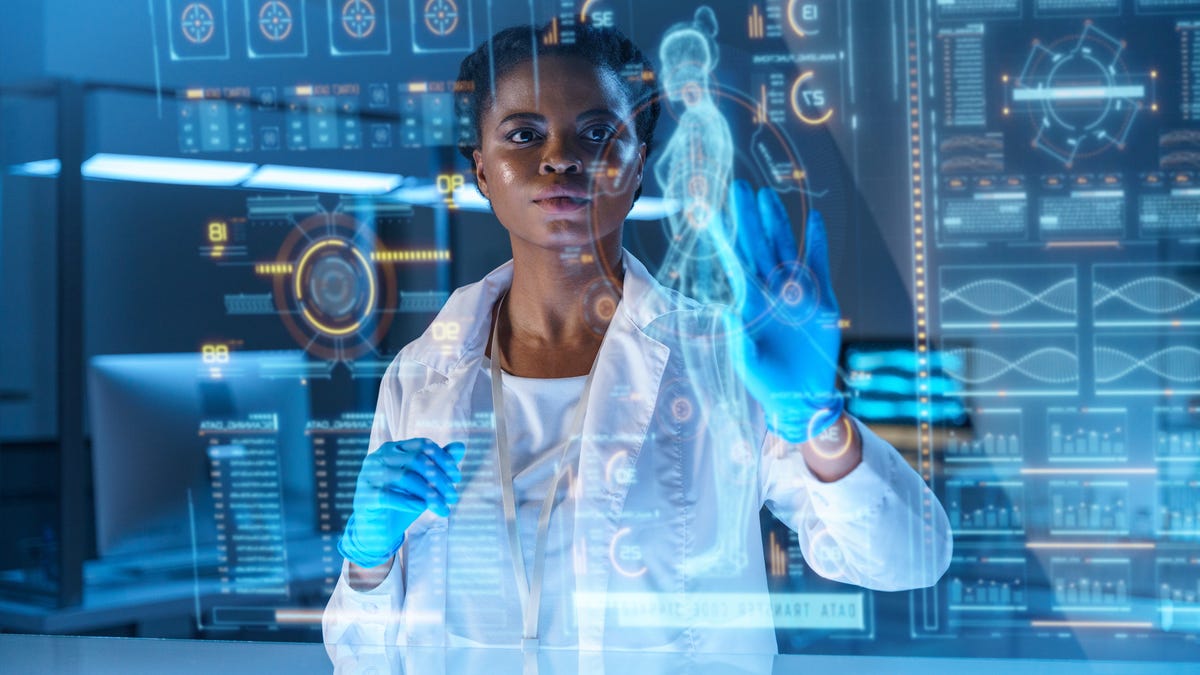

Most functions of synthetic intelligence in drugs have didn’t make use of language, broadly talking, a undeniable fact that Google and its DeepMind unit addressed in a paper printed within the prestigious science journal Nature on Monday.
Their invention, MedPaLM, is a big language mannequin like ChatGPT that’s tuned to reply questions from a wide range of medical datasets, together with a model new one invented by Google that represents questions customers ask about well being on the Web. That dataset, HealthSearchQA, consists of “3,173 generally searched shopper questions” which are “generated by a search engine,” akin to, “How severe is atrial fibrillation?”
Additionally: Google follows OpenAI in saying virtually nothing about its new PaLM 2 AI program
The researchers used an more and more necessary space of AI analysis, immediate engineering, the place this system is given curated examples of desired output in its enter.
In case you have been questioning, the MedPaLM program follows the latest development by Google and OpenAI of hiding the technical particulars of this system, relatively than specifying them as is the usual follow in machine studying AI.
Google’s MedPaLM builds on prime of a model of its PaLM language mannequin, Flan-PALM, with the assistance of human immediate engineering. Google/DeepMind
The MedPaLM program noticed a giant leap when answering the HealthSearchQA questions, as judged by a panel of human clinicians. The proportion of occasions its predictions have been in accord with medical consensus beat the 61.9% rating for a variant of Google’s PaLM language mannequin, reaching 92.6%, simply shy of the human clinician’s common, 92.9%.
Nevertheless, when a bunch of laypeople with a medical background have been requested to fee how effectively MedPaLM answered the query, that means, “Does it allow them [consumers] to attract a conclusion,” 80,3% of the time MedPaLM was helpful, versus 91.1% of the time for human physicians’ solutions. The researchers take that to imply that “appreciable work stays to be completed to approximate the standard of outputs offered by human clinicians.”
Additionally: 7 superior prompt-writing ideas it is advisable know
The paper, “Massive language fashions encode scientific data,” by lead creator Karan Singhal of Google and colleagues, focuses on utilizing so-called immediate engineering to make MedPaLM higher than the opposite massive language fashions.
MedPaLM is a by-product of PaLM-fed question-and-answer pairs offered by 5 clinicians within the US and UK. These question- reply pairs, simply 65 examples, have been used to coach MedPaLM through a collection of immediate engineering methods.
The everyday method to refine a big language mannequin akin to PaLM, or OpenAI’s GPT-3, is to feed it “with massive quantities of in-domain knowledge,” observe Singhal and staff, “an strategy that’s difficult right here given the paucity of medical knowledge.” As an alternative, for MedPaLM, they depend on three prompting methods.
MedPaLM considerably outperforms Flan-PaLM in human evaluations, although it nonetheless falls in need of human clinicians’ skills. Google/DeepMind
Prompting is the follow of bettering mannequin efficiency “by way of a handful of demonstration examples encoded as immediate textual content within the enter context.” The three prompting approaches are few-shot prompting, “describing the duty by way of text-based demonstrations”; so-called chain of thought prompting, which includes “augmenting every few-shot instance within the immediate with a step-by-step breakdown and a coherent set of intermediate reasoning steps in direction of the ultimate reply”; and “self-consistency prompting,” the place a number of outputs from this system are sampled and a majority vote signifies the fitting reply.
Additionally: Six abilities it is advisable grow to be an AI immediate engineer
The heightened rating of MedPaLM, they write, reveals that “instruction immediate tuning is a data-and parameter-efficient alignment approach that’s helpful for bettering elements associated to accuracy, factuality, consistency, security, hurt, and bias, serving to to shut the hole with scientific specialists and convey these fashions nearer to real-world scientific functions.”
Nevertheless, “these fashions should not at clinician skilled stage on many clinically necessary axes,” they conclude. Singhal and staff recommend increasing using skilled human participation.
“The variety of mannequin responses evaluated and the pool of clinicians and laypeople assessing them have been restricted, as our outcomes have been primarily based on solely a single clinician or layperson evaluating every response,” they observe. “This could possibly be mitigated by inclusion of a significantly bigger and deliberately various pool of human raters.”
Additionally: write higher ChatGPT prompts
Regardless of the shortfall by MedPaLM, Singhal and staff conclude, “Our outcomes recommend that the robust efficiency in answering medical questions could also be an emergent capacity of LLMs mixed with efficient instruction immediate tuning.”
Unleash the Energy of AI with ChatGPT. Our weblog offers in-depth protection of ChatGPT AI know-how, together with newest developments and sensible functions.
Go to our web site at https://chatgptoai.com/ to study extra.