Harness the Potential of AI Tools with ChatGPT. Our blog offers comprehensive insights into the world of AI technology, showcasing the latest advancements and practical applications facilitated by ChatGPT’s intelligent capabilities.
A team of researchers from UC Berkeley, UCL, CMU, and Google Deepmind address the challenge of optimising large language models using composite reward models derived from various simpler reward models. These hybrid models often need help with the appropriate weighting of component models, leading to over-optimization, where higher reward correlates with worse human ratings. Their method proposes a solution using constrained reinforcement learning to prevent the agent from exceeding each component model’s usefulness threshold.
The study refers to a vast history of research on integrating constraints into reinforcement learning. It mentions studies by authors such as Borkar, Padakandla, Cheung, Lecarpentier, and more. The study also highlights the importance of addressing non-stationarity in reward functions and cites works by Moskovitz, O’Donoghue, and Tarbouriech. Moreover, the study discusses the use of regularised policy optimization.
LLMs excel in natural language processing but face issues with safe deployment and alignment with human preferences. Reinforcement Learning from Human Feedback (RLHF) adapts LLMs using reward models that mimic human choices. However, over-optimization of RMs can lead to poor text quality. Their work suggests a solution with composite reward models, addressing over-optimization by identifying proxy points and using constrained optimization. Dynamic weighting controls each RM’s influence on the learning process.
The analysis introduces constrained reinforcement learning using Lagrange multipliers to manage over-optimization in composite reward models. It enforces constraints on component reward models, keeping them within the effective human evaluation range. An adaptive gradient-free optimization method is presented to identify and optimise proxy points to prevent reward model overuse. Different task reward and constraint threshold formulations, including KL divergence, are considered.
Their approach conducts the first study on over-optimization in composite reward models, revealing a correlation’s significant impact on over-optimization points. An adaptive gradient-free optimization method is employed to prevent exceeding reward model thresholds. PPO algorithms, including PPO-SAT and All-PPO, are discussed for implementing constrained reinforcement learning. Detailed pseudocode is provided, covering various task reward and constraint threshold formulations.
The research focuses on solving optimization challenges in composite reward models that affect language quality evaluation. An adaptive gradient-free optimization method is used to identify and optimise over-optimization points. The study delves into implementing PPO algorithms such as PPO-SAT and All-PPO. It emphasises the significance of appropriate weighting and correlation consideration among component reward models for effective language quality evaluation.
Future research should consider applying reliable approaches like ReLOAD to tackle over-optimization in composite reward models. Exploring the utility of CMDP formulations to prevent model output issues in cases without deterministic optimal policies is essential. Extensive testing across diverse domains and complex composite reward models is warranted. Investigating alternative reinforcement learning methods and evaluating the influence of weighting strategies and correlation measures on the proposed approach’s performance is crucial for further advancements.
Check out the Paper. All Credit For This Research Goes To the Researchers on This Project. Also, don’t forget to join our 32k+ ML SubReddit, 40k+ Facebook Community, Discord Channel, and Email Newsletter, where we share the latest AI research news, cool AI projects, and more.
If you like our work, you will love our newsletter..
We are also on WhatsApp. Join our AI Channel on Whatsapp..
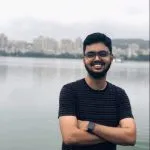
Hello, My name is Adnan Hassan. I am a consulting intern at Marktechpost and soon to be a management trainee at American Express. I am currently pursuing a dual degree at the Indian Institute of Technology, Kharagpur. I am passionate about technology and want to create new products that make a difference.
Discover the vast possibilities of AI tools by visiting our website at
https://chatgptoai.com/ to delve deeper into this transformative technology.
Reviews
There are no reviews yet.